How to Modernize Traditional Data Transformation: A Look at Cloud ELT
In 2021, enterprises are looking for faster and more efficient ways to transform and prep mountains of data. Matillion’s Laura Malins describes how Cloud ELT empowers data prep for faster insights, machine learning support and more.
by Laura Malins, product manager, Matillion
Tags: analytics, cloud, data, ELT, ETL, Matillion, machine learning, transform,
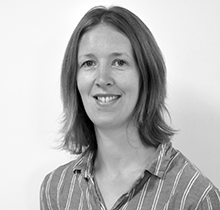
product manager
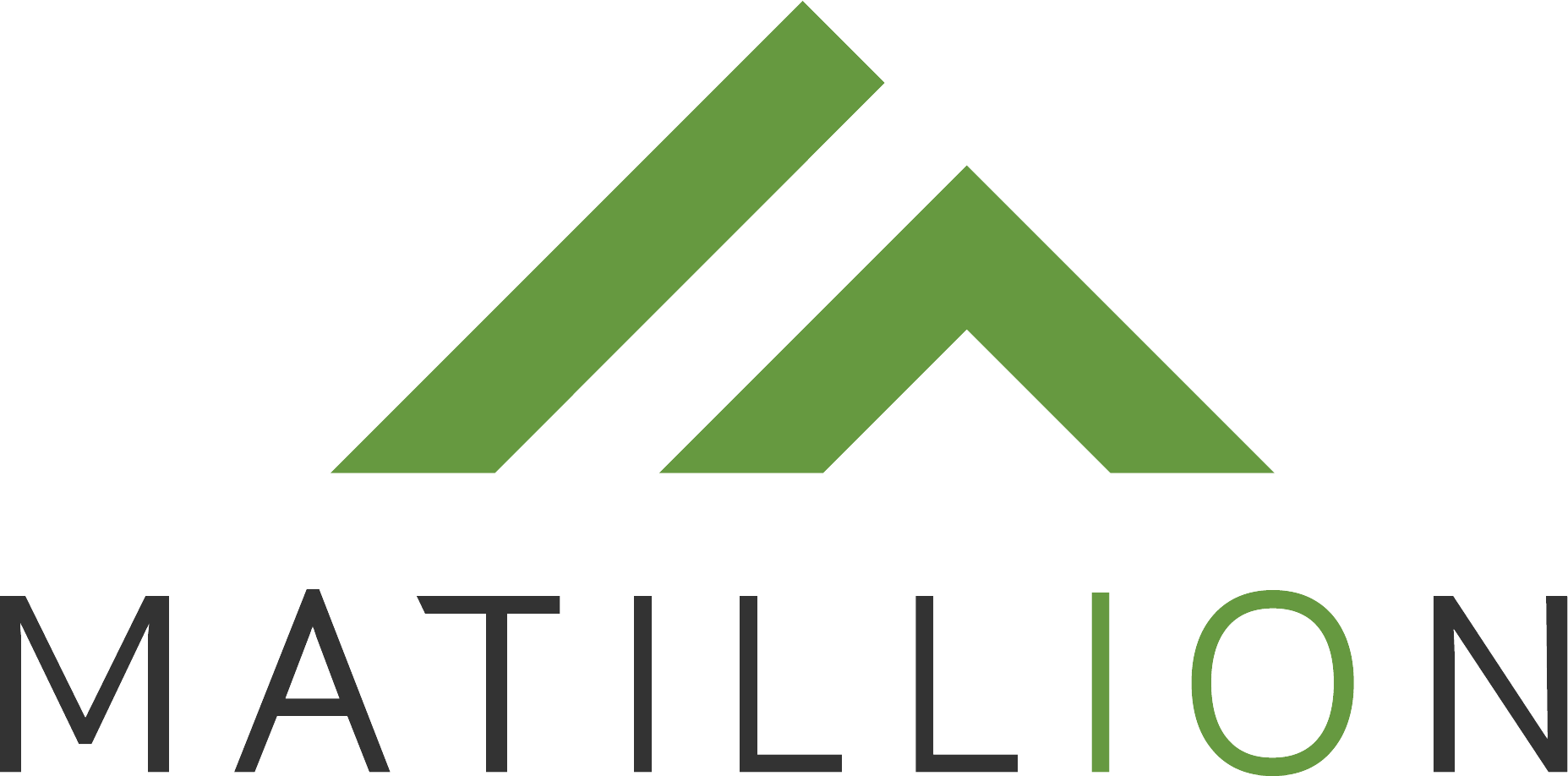
"Modern data technologies need to not only move data into the cloud -- but also support analytic requirements."
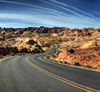
Most companies create massive amounts of data in all areas of their business. But what makes a competitive difference is whether they can leverage that data to predict outcomes, reduce costs and inefficiencies, increase revenue and better the customer experience - thus giving it tangible business value.
To achieve any of these beneficial outcomes, companies must be equipped to mine their data for insights.
In other words, modern data technologies need to not only move data into the cloud -- but also to manipulate and transform it to support downstream analytic requirements.
A modern approach to data transformation is achieved using Cloud ELT (extract, load, transform -not to be confused with traditional ETL: extract, transform, load). Cloud ELT can harness the extraordinary scale and speed of cloud data warehouses to power large scale data transformation in minutes instead of weeks or months. Cloud ELT is the process in which the transformation is done using the cloud environment's power and performance. The result: Enterprises obtain the ability to operationalize data, transforming organizations into data-driven leaders of the future.
The remainder of this article will review some of the more impactful benefits of a Cloud ELT approach.
Cloud-Ready Data Transformation
Data transformation is the ability to join together siloed data, denormalize, enrich, and apply business logic – and it is crucial to reveal insights that will help a business pull ahead. But it isn't easy. Ninety percent of enterprises say that making data available for insights is a key challenge in their analytics programs.
When performed in the cloud, data transformation can help organizations rapidly improve data management and existing processes. Analytics-ready data can help solve critical business problems and ultimately generate revenue. As external factors contribute to pressure on budgets, resources, and roadmaps, transforming data with an ELT solution will help reduce development time and accelerate innovation with new insights.
Modernizing traditional data transformation through Cloud ELT can help enterprises reduce costs, centralize data sources for easier reporting and access, scale data transformation workflows and processes, and prepare data for machine learning models.
Faster Time-to-Insights
As companies look to improve their data-driven culture, the need for fast and modern data integration is paramount. With the explosion of new and varied data sources constantly streaming inside large enterprises, moving quickly to prevent data from becoming stale can be a challenge. Companies with older data architectures are unprepared for this variety and scale and can face big barriers to achieving analytics-ready data sets.
Cloud data transformation empowers employees with timely data that allows them to innovate faster, decrease the time required to create critical revenue metrics, decrease the need for custom code and reduce costly administration for data warehouses and ETL/ELT.
Enterprises that adopted cloud data transformation saw significant reductions in their reporting time, going from over six hours to just 30 minutes in some instances.
Cost Savings
Replacing an aging data architecture with a modern cloud-native solution is cost-effective, provides faster time to value and is scalable. A data warehouse that is procured and provisioned in the cloud can solve some of the most difficult challenges plaguing on-premises data stacks: poor performance and high costs when dealing with increased volumes of data and proliferating siloes across the organization.
With a modern data architecture that includes cloud-native data integration and transformation solutions, enterprises are proactive, competitive, and agile in light of new data insights— all while saving money.
Centralizing Data Sources for Easier Reporting
Every department within an organization has data that needs to be collated, transformed and then analyzed. For sales forecasting, marketing operations, financial reporting, this data should all live in a central place for easy access and accurate reports. A single view of data can help companies understand the importance of their data and its lineage. Cloud ELT solutions easily migrate data from source systems into a cloud data warehouse where it can be accessed and processed quickly.
A series of jobs can be configured to join together the separate data and embellish it with metrics and business logic for easy-to-understand, rapid reports on an hourly or daily basis. With this approach, reusable components and frameworks to extract data from numerous sources can save development time and reduce the data ingestion process from as many as ten workflows down to just one.
This is much more attractive than having to manually collate and process the data, which can take so long that reports can only be available weekly or monthly.
Data Preparation for Machine Learning
Cloud-native data transformation enables organizations to power AI/ML projects with trusted data from more sources in less time – generating faster, more reliable results that drive previously unrealized business value and innovation.
Traditionally, data professionals spend so much time gathering and validating data to prepare it for use. They have little time left to focus on their primary objective - analyzing that data and deriving business value from it.
When advanced analytics such as machine learning and artificial intelligence are introduced, the volume of data required makes this task even more daunting and likely to take over the job.
Current data preparation efforts such as data wrangling and traditional ETL require manual effort from IT professionals who struggle to keep up with big data initiatives' scale and complexity. For example, a global logistics firm making 100 million deliveries to 37 million unique customers in 72 countries needs vast amounts of data to power its daily operations. Using cloud data transformation enabled the company to deploy 200 machine learning models in a single year. These models make 500,000 predictions every day, significantly improving efficiency and driving superior customer service that has reduced inbound call center calls by 40 percent.
Modern Data Transformation Drives Faster Data Innovation
Companies are taking advantage of agile, modern cloud technology by blending cloud and hybrid data management architectures. A recent study showed that more than one-third of enterprises are already using cloud data warehouses and 43 percent expect to have all of their data in the cloud. The remainder is planning to pursue hybrid models that leverage both cloud and on-premises data warehouses.
The Time for Cloud ELT is Now
The technology to modernize data transformation – and achieve all these benefits - exists today with the power to propel a business into the future by enabling insights that guide decision-making. By employing the cloud for its many benefits, an organization will improve data management and other processes and gain effective analytics faster to drive innovation.
Cloud ELT provides the speed and scale to make data useful for enterprises due to its flexibility and powerful performance. With data transformed and ready for analytics, the ability to become a truly data-driven business is more of a reality than ever before.
Laura Malins is a Product Manager at Matillion, the leading provider of data transformation for cloud data warehouses (CDWs). She has 15 years of experience in the world of data, spanning a variety of different technologies and industries including finance and consultancy.
Related:
- The Critical Integration Miss: Continuous Exploration
- Survey: 92% of Enterprises Working on, Planning App Modernization
- Tray.io Low-Code Integration, Automation Updates Drive Enterprise Hyperautomation
- Matillion Adds No-Code, Automation To Speed Delivery of CDC and Batch Data Pipelines
- Tray.io 'Embedded iPaaS' Empowers SaaS, ISVs via Low-Code, Automated Integrations
All rights reserved © 2024 Enterprise Integration News, Inc.