Can AI Transform Application Testing?
According to app store giants Google and Apple, 80% of downloaded apps are only used once -- and a whopping 96% aren’t used after the first month. With such meager success rates for new apps, Dr. John Bates, CEO at TestPlant, explores the question: Can AI transform app testing – and bring us all better results?
by Dr. John Bates, CEO TestPlant
Tags: AI, algorithms, apps, bugs, continuous, DevOps, intelligence, QA, testing, TestPlant,
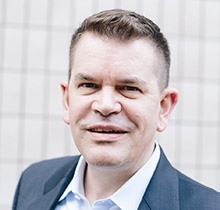
CEO
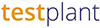
"Testing apps is no longer just about does it work as specified? Today, testing is about ‘Will it delight customers?’"
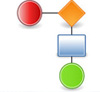
According to app store giants Google and Apple, 80% of downloaded apps are only used once and 96% aren’t used after the first month. To look for the culprit for such disappointing results, one need only look for the strong (if not direct) correlation between user experience, retention, revenue, and app performance.
So, in this light, no wonder that app testing is taking on new importance. Testing is no longer just about “does it work as specified?” Today, testing is becoming about “will it delight customers” and provide a high quality, performant and usable ‘digital experience’? This shift means digital teams are currently struggling to deliver high-quality digital experiences that delight users in a way that’s fast and engaging.
Many experts today say that artificial intelligence (AI) is in a position to fundamentally change the way we work and live. In fact, during Google's I/O conference last May, the company stated that we already live in an AI-first world -- and no one can escape the hype.
So, the question naturally arises: What might AI mean for how we develop and deliver software and applications? Can AI actually improve the odds of building a successful (and delightful) app?
It turns out, in testing and monitoring of the digital experience, AI and analytics can be critical – but perhaps not in the way you may be thinking.
There’s a lot of talk currently about test automation. However, in reality, we’ve only automated one key element: test execution. AI and analytics will be the catalysts to deliver true test automation that recommends the tests to carry out, learns continuously, enabling it to predict business impacts, and enabling dev teams to fix issues before they occur.
So, here are three ways we see AI is enabling this new type of ‘predictive’ testing:
1. Intelligent automation - The only way to realistically test a digital app is through an intelligent automation engine accessing the application as a user would - taking control of a machine, actually using the app to exercise workflows and collecting intelligent analytics along the way. This involves technology to understand on-screen images and text, such as smart image search and dynamic neural networks (so called “deep learning”).
2. Intelligent test coverage generation and ‘bug-hunting’ - There are a potentially infinite number of paths through a complex app so which ones should we follow in our automation? We can use AI classification algorithms such as Bayesian networks, to select paths and 'bug hunt'. As these paths are explored, the bug-hunting AI algorithm continues to learn from correlations in data to refine the coverage and help developers identify root causes and fix defects.
Through a combination of bug hunting and coverage algorithms, AI and analytics will exponentially increase coverage and productivity. AI algorithms will hunt for defects in applications based on user journeys automatically generated from this bug-hunting model, while coverage algorithms will select the user journey that is the furthest away from others that have already been executed. Non-instance-based learning algorithms also reduce the amount of 'learning' required giving quick results, essential in Agile and DevOps environments. Ensuring that the algorithms are delivering defects and coverage is a balancing act, and where AI will need input from a smart tester who knows the system well and can dynamically adjust the trade-off between coverage and bug-hunting.
3. Continuous test, continuous learning, predictive trends - Testing digital apps is not just a ‘one and done’ exercise. It should be a continuous process - so that we are essentially monitoring the digital experience over time. And an AI algorithm should be watching the test results over time - learning and looking for trends. These learning algorithms can then build decision trees that enable predictive analytics to identify, for example, if based on experience, the increasing delay on a particular workflow, we’re heading for a system outage. We could address this before it becomes critical and causes customer outrage.
The transformative impact of AI and analytics will go even further to fundamentally change what testing is about, bringing the user and their satisfaction directly into testing. Testing needs to focus on the user if it’s going to improve the experience. Today, the success of digital apps are measured in terms of net promoter score, responsiveness, usability and reliability. Automation algorithms that simulate the way applications are deployed across different devices and networks, with different workflows and parameters running all the time, will provide a view of what any user would be seeing — so the quality experience can be dramatically improved.
Testing will move closer to the user and the product designer through predictive analytics recommendations. By learning the relationship between technical behaviors and user satisfaction/conversion/retention, testing will ultimately move closer to revenue. In just a few years, AI will ensure that testing is seen as a revenue-generating profit center that increases customer conversion and retention, rather than an unwieldy compliance overhead.
So, now the next obvious question: Will these smart algorithms replace humans anytime soon?
In my opinion, No. That said, intelligent test automation offers key advantages that will drive productivity of the human tester. AI will also likely increase testers’ ability to discern what makes for a delightful app.
AI, algorithms will evaluate systems to auto-generate previously resource intensive scripts, analyze the results to predict bugs and adjust the scripts to improve test coverage.
For all these benefits of AI-driven full test automation, we’ll always need testers and business analysts. Humans will be the ones to set the objectives and the parameters of automation, guide AI learning, and add intent and human interpretation. As Eric Siegel stated in the Induction Effect: “Art drives machine learning; when followed by computer programs, strategies designed in part by informal human creativity succeed in developing predictive models that perform well on new cases.”
In summary, to achieve the goal of automation in testing the digital experience, you need tools that can intelligently navigate applications, predict where the quality issues are most likely to be, and identify the key data correlations that will help developers resolve issues quickly. AI will transform app dev, and, in turn, have a dramatic impact on user adoption, conversion, retention and—most critically—revenue generation.
Dr. John Bates is CEO of TestPlant. A visionary technologist and accomplished business leader, John holds a Ph.D. in Computer Science from Cambridge University, UK and is the author of the book “Thingalytics: Smart Big Data Analytics for the Internet of Things”. Follow John at @drjohnbates.
Related:
- Salesforce Partner Conga Turns to AI/ ML For Smart End-to-End Documents & Contract Management
- With Expanded Machine Learning Capabilities Across Portfolio, Splunk Unveils New Use Cases
- Electric Cloud Delivers Rich Analytics for DevOps; Correlates Data and Views from Multiple Tools
- Riverbed Improves ‘Digital Experience Management’ with Latest SteelCentral Update
- DataDog Monitoring Integrates with AWS CodeDeploy To Help Developers More Easily Locate, Resolve Faulty Deployments
All rights reserved © 2024 Enterprise Integration News, Inc.