Coming and Current AI Regulation: Four Ways Scalable Model Ops Can Help Readiness
Even as enterprises are expected to invest multiple millions in AI initiatives, many continue to overlook two key aspects – trust and transparency. TIBCO’s Lori Witzel shows why these two elements should be part of every AI initiative in 2022, along with tips on the best way to start and succeed.
by Lori Witzel, Director of Research for Analytics and Data Management at TIBCO
Tags: AI, analytics, explainability, machine learning, MLOps, trust, transparency, regulations, risks,
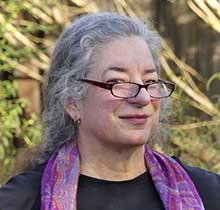
director, research
analytics & data management
"Increasing understanding of AI and machine learning and your involvement with oversight and governance will help ensure your company reaps the most risk-free rewards."
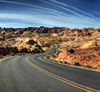
AI is a hot topic, and it’s often associated by the public with threats to job security and social displacement. As organizations seek to use AI for operational excellence, improved customer experience and intimacy, or business reinvention, building trust and transparency into AI is a core best practice.
By requiring that trust and transparency are part of AI innovation, everyone benefits.
Businesses can reap AI’s rewards, and the public can be assured that privacy and ethics are part of AI initiatives. Business leaders have an important role to play in this – especially as new AI regulations are drafted globally.
Why “Trust and Transparency” Now?
At both Federal and state levels, US government agencies are turning their attention to managing potential risks related to AI. The Federal Trade Commission’s April 2021 blog, “Aiming for truth, fairness, and equity in your company’s use of AI,” indicates the FTC will use its authority to pursue the use of biased algorithms. The FTC’s ongoing enforcement actions show they’re serious about pursuing compliance for AI fairness and transparency. As they wrote, it’s time to “Hold yourself accountable – or be ready for the FTC to do it for you.”
States are also engaged in their own AI bills and resolutions. Nearly 20 states introduced bills or resolutions in 2021, and four states enacted them to date.
If that wasn’t enough to make businesses take notice, those with customers or business partners in the EU will soon have to adhere to the European Union Artificial Intelligence Act (EU AI Act), which is in draft. At its core, the Act is intended to ensure humans are at the center of AI innovation and governance.
The impact of the EU AI Act is likely to be as wide-ranging as GDPR is, and like GDPR, the timeline might seem generous, but even US businesses need to begin preparing.
What To Know—and What To Do—about AI Trust and Transparency
Trustworthy AI systems are transparent and auditable. Transparent AI systems are built to ensure traceability, explainability, and clear communication of AI models, algorithms, and machine learning processes. Not only should there be a path to describing the processes, tools, data, and actors involved in the production of the AI models, it also means clearly defining the intent behind and selection process for use cases.
Auditable AI systems require models and documentation to be clear enough that auditors could produce the same results using the same AI method based on the documentation made by the original data science team. Think of this as a form of reverse engineering to prove transparency.
Understanding the Relationship Between Trust and Machine Learning Models?
Data scientists experiment with many different datasets, upon which many different machine learning models are configured and applied. These experiments often require varying one parameter and holding others constant. They do so to identify a model that, with a given set of parameters, produces desired results on a selected dataset.
Transparency and trust require being able to understand a model’s lineage—the set of associations between a model and all the components involved in its creation. Without robust, scalable model operations, tracking a model’s lineage may not be complete, or even possible. And as you can see from the process described, the number of components can be large, dynamic, and difficult to trace.
The diagram below shows where business leaders and stakeholders should be involved to support improved trust and transparency.
Both trust and transparency can be addressed with robust, scalable model management and model operations. Model operations—the development and management of machine learning models that support AI initiatives—is key to operationalizing AI, and can be difficult to scale.
Four Ways Scalable Model Operations Can Help Your Regulatory Readiness
The right model operations tooling, in combination with human oversight, governance processes, and de-biased training data, will help:
Support transparency. Model management helps ensure that machine learning models are traceable and explainable. Transparency and trust require being able to understand a model’s lineage.
Enable auditability. By bringing machine learning models into model operations processes, audit paths will be clearer. Model management means more facets of a model’s lineage will be traceable and reproducible, important for regulatory transparency.
Make AI at scale possible. Model deployment at scale is an obstacle adding friction to the path for AI value. Those adopting best-in-class model management tools report increasing model deployments from tens to thousands of models, enabling those organizations to leap ahead of competitors in the race to get value from AI.
Reduce risk. With transparent, auditable machine learning model management, risks from adverse AI impacts and regulatory risk are decreased. If inadvertent harms from AI occur, they can be quickly traced back and remediated.
Automating model management at scale, with tooling that provides support for governance and oversight, is not an option if you want to realize the promise of AI while planning to meet regulatory compliance needs.
Recommendations: Next Steps for Business Leaders and Stakeholders
To support trustworthy, transparent, compliant AI:
- Engage with data science and data management teams to discuss current and future AI initiatives.
- Familiarize yourself with the FTC’s notes regarding AI and the coming EU AI Act, and make sure that business stakeholders are familiar with current and coming regulations.
- Collaborate with your organization’s legal team—those who were involved in GDPR planning, for example. Ensure they have visibility through AI workgroups or an AI Center of Excellence, given the legal dimensions of increased AI regulation.
- Ask AI and IT teams to share their perspective on model management and deployment, and work together to enable AI at scale.
Above all, stay engaged. AI and machine learning are already transforming our world in positive ways—for example, AI’s role in speeding the creation of an effective mRNA COVID-19 vaccine for clinical trials.
Increasing your understanding of AI and machine learning and your involvement with oversight and governance efforts will help ensure your company reaps the rewards of AI while managing regulatory and compliance risks.
Lori Witzel is Director of Research for Data Management and Analytics at TIBCO. She develops and shares perspectives on improving business outcomes through digital transformation, human-centered artificial intelligence, and data literacy. Providing guidance for business people on topical issues such as AI regulation, trust and transparency, and sustainability, she helps customers get more value from data while managing risk. Lori collaborates with partners and others within and beyond TIBCO as part of TIBCO’s global thought leadership team.
Related:
- Cinchy Dataware Platform Expands Scalability; Adds Support for Kubernetes, PostgreSQL
- Actian Enhances Data Quality, Automation and Self-Service for Data Integration
- Tibco's New Hyperconverged Analytics Approach Delivers Rapid, Actionable Insights for Customers
- Self-Service is the Future And It’s Time To Embrace It
All rights reserved © 2024 Enterprise Integration News, Inc.